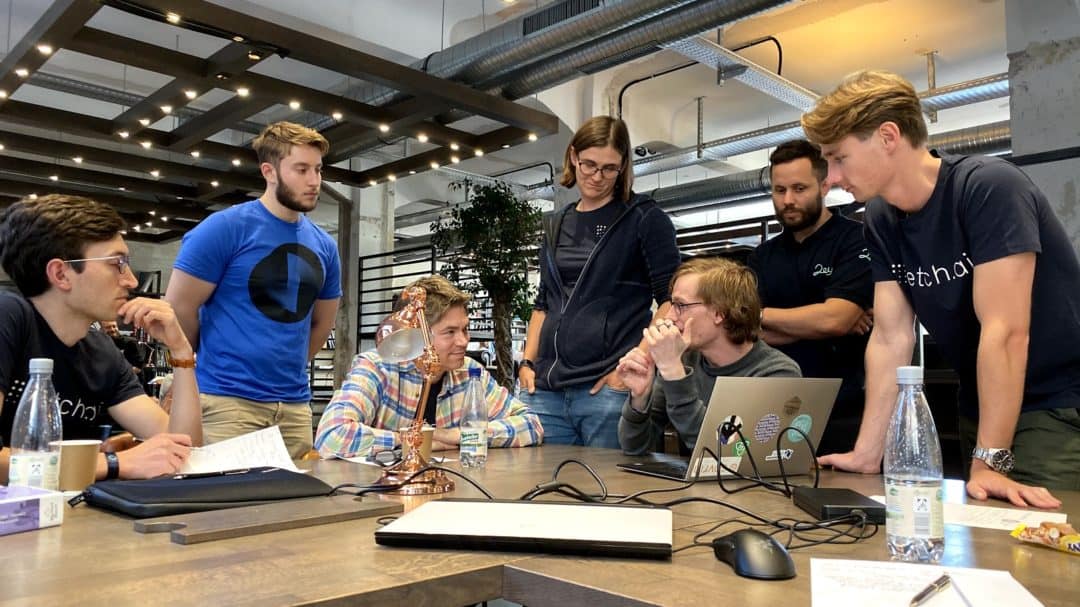
For the last year, we at Datarella have been working together with two leading sensor, IoT and infrastructure providers to collectively set technological standards and build distributed ledger technology to shape the future of mobility. On 19th and 20th of October Datarella participated at the Diffusion Hackathon by Outlier Ventures to experience live the 20 most exciting Web 3 protocols. We had the opportunity to dive deep into the Fetch.ai tech stack during the event and were able to leverage those tools to build a PoC over the course of two days. Fetch offers a unique agent-based approach to allow developers to turbocharge the matchmaking capabilities enabled by converging blockchain and AI. We proudly announce that our team of Rebecca Johnson (Datarealla), Tom Rae (Agent3), Idan Portal (2key), Tomasz Gęsior (Baltic Data Science) and Philipp Kothe (Datarella) won with our project “Effortless Parking” in two tracks.
During the hackathon, we built a project on top of a real-world use case in development within our consortium. Our Diffusion 2019 project is meant to extend and build on top of our current prototypes for improving coordination between parking lot operators, cars and the IoT & infrastructure providers whose systems make up the mobility landscape in which parking transactions take place.
Our goal was to increase the efficiency in the parking market by reducing the transaction costs which occur between the driver and the parking lot owner. Further, we wanted to prove that an open and collaborative market, enabled by web3 technologies, would be beneficial for both sides of this market. To strengthen our argument we will refer in this post to two economic theories, the transaction cost, and economic surplus theory.
1. Transaction costs
According to a study, car drivers spend around 40 hours a year searching for a parking space. This is not only a waste of time but is also responsible for one-third of all traffic, respectively traffic-related air pollution in inner cities. At the moment drivers have to download multiple apps and compare the offers of various parking garage operators to evaluate what is the best parking option close to the destination. Alternatively, many drives just drive around looking visually for an opportunity to park. However, both approaches are related to high opportunity and searching costs for the consumer.
These costs together are also referred to as transactions costs and occur whenever a good or service is transferred via a technologically separable interface. Transaction costs describe both monetary and indirect costs such as time or effort. For a systematic approach, these costs can be divided by the time they take place in the transaction process. For example, prior to the conclusion of a contract, time needs to be spent searching and evaluating information, negotiating price and drafting/reviewing contracts, adjusting the contract/s, and finally, after contract execution, time must be spent to resolve any post-settlement disputes.
Like all costs, transaction costs have a limiting effect on economic growth. As a result, technological and organizational innovations that reduce transactions costs for users are becoming increasingly important for macroeconomic development.
2. Economic Surplus
Also, on the other side of the market, we identified high inefficiencies. In contrast to airlines or hotels, car park providers currently do not usually implement any kind of capacity utilization-based pricing. This, in combination with a lack of coordination and information among drivers, leads to a system of suboptimal asset utilization since some car parks are overbooked and while others are still nearly empty. The consequence is a limitation of the economic surplus created in the parking industry.
Economic surplus is a macroeconomic concept established and framed by the economist Alfred Marshall in the mid-19th century. Together with Karl Heinrich Rau, he developed a diagram, which shows the relation of demand and supply in dependency on price and available quantities of an economic good.
But let’s take this into practice and look at an example. The paid parking market in Munich consists of over 24 parking garages which offer about 7400 parking spaces.
In this simplified example, three scenarios are possible as shown in the graphic.
1. If the price is too high, there is not enough demand and we face a surplus in parking spaces.
2. If the price is too low a shortage occurs because there would be more people willing to park than operators offering lots.
-> In both cases, a deadweight loss is created and value is lost since the full market potential cannot be captured.
3. The equilibrium price is found. Hereby, the price is set where demand exactly meets supply, which allows to capture the full market potential. Important to note is that this system is referring to the average price, which is composed of the individual prices of each parking garage.
3. The Fetch.ai Solution
To solve this dilemma of coordination and optimal pricing our team used Fetch.ai, a system designed to increase market efficiency by helping to match supply and demand.
So, during this hackathon, we used Fetch.ai to build a software simulation that would allow us to model the revenue generated by the parking providers under both optimal and suboptimal coordination conditions. Our first model simulated the revenue of each parking lot operator without any cooperation between the different provider, coordination or dynamic capacity utilization based pricing. In that model, drivers just attempt to find the closest spot (which is close to the real behavior of inner-city drivers). The second model simulates a smart network in which the different operators collaborate and drivers are coordinated to the available parking spaces, which match their preferences for distance and price. Autonomous economic agents powered by AI negotiate to achieve economically efficient outcomes.
We implemented our project using Fecht.AI prebuild SDK library, which allowed us to run a local ledger and a local IEF node. Further, we implement a custom data service to represent the parking garages. In this model, the parking garages acted as data provider and the drivers compared then the data they saved as preference vector (like distance to destination, price..) with all of the available data from the garages. After all options are evaluated, the agents choose the best option for the driver and sets a deposit in Fetch (FET), the native token of the ecosystem, to reserve the parking spot. We then used Fetch.ai smart contracts to represent the tickets which are sold and bought.
This system allows to discover the equilibrium of the whole parking market dynamically, reacting to any changes in capacity utilization in real time. Practically it can be used to demonstrate to parking lot operators that they are leaving significant money on the table by not using a fair and neutral DLT based coordination and booking system alongside their competitors. Everyone’s better off in this model as deadweight loss and negative externalities are removed from the system while maximizing both parking operator revenues and net economic surplus.
We are absolutely thrilled to further discover the endless possibilities Fetch.ai offers. The best part of the system is the fact that these “simulations” are intended to be implemented within production systems in the future. This means that the same system we’re using to simulate the future economic and environmental effects can then be put into use to actually achieve those outcomes in a real-world system.